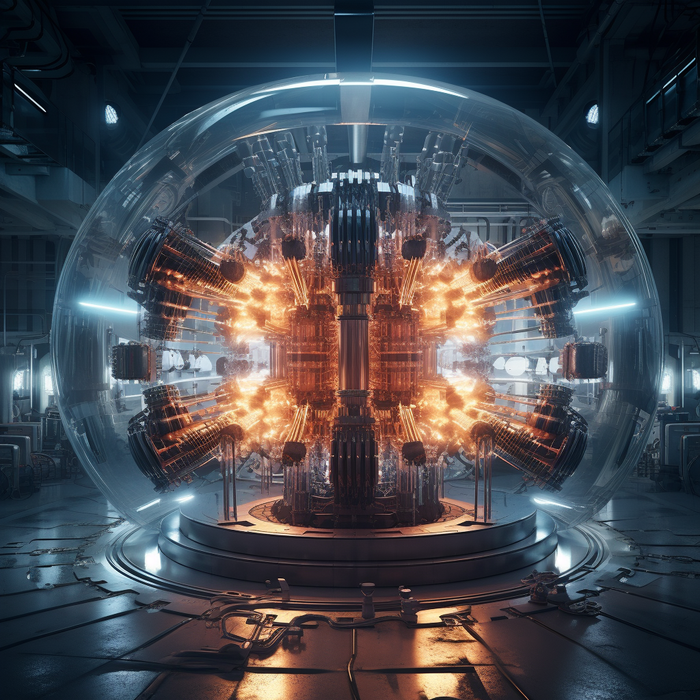
AI generated Tokamak by natti@dr-perez-rivas-consulting.com
In this blog, we explore the transformative potential of artificial intelligence (AI) in advancing fusion energy. By leveraging AI-driven simulation techniques, researchers are unlocking new possibilities and accelerating progress in the field of fusion energy. Discover how AI is revolutionizing the way we approach fusion energy, leading us towards a future of clean and abundant energy sources.
Introduction
The image above depicts ITER a multinational collaborative project situated in southern France, which strives to showcase the viability of fusion energy on a large scale. With the participation of 35 countries, ITER stands as a significant endeavor currently undergoing construction, bringing together global expertise and resources to advance the frontiers of fusion energy research.
In recent times, fusion energy has experienced a resurgence of interest, particularly from private companies that are eagerly investing in this transformative technology. The construction of the ITER magnetic confinement fusion device, coupled with significant breakthroughs, has opened up numerous possibilities for leveraging machine learning (ML) to enhance simulations and maximize the efficiency of these devices. While fusion energy has a rich history of research and development, the recent strides made in this field have reignited enthusiasm and instilled a renewed sense of promise for a future powered by clean and limitless energy.
What is Fusion Energy?
Fusion energy is a type of energy generated by the fusion process (showed above), which occurs when two atomic nuclei come together to form a heavier nucleus, releasing an immense amount of energy in the process. This phenomenon is the same process that powers the sun and other stars in the universe.
There are numerous fusion reactions that can be considered for energy production. One of the easiest fusion reactions to achieve on Earth is the reaction between deuterium (D) and tritium (T).
This reaction can be expressed as: D + T -> He + n + Energy (17,699 keV)
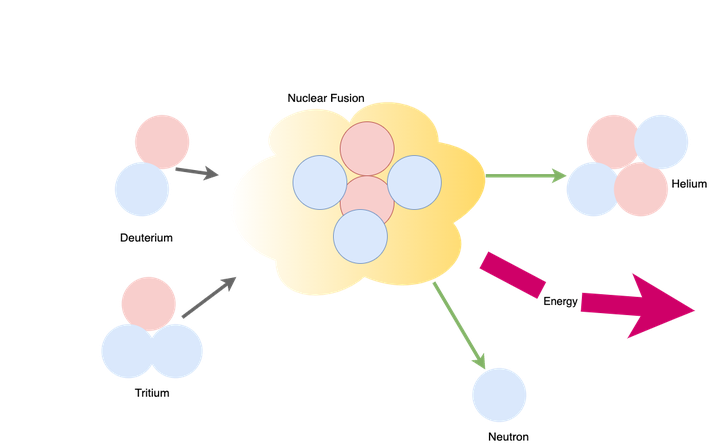
In this reaction, deuterium and tritium combine to form helium (He), a neutron (n), and release a significant amount of energy. The energy production from this fusion reaction is significant. The maximum probability energy release is around 70 keV, but the total energy release is much higher at 17,600 keV. This translates to an energy production that is approximately 250 times greater than the input energy. To put it into perspective, the energy release from this fusion reaction is 4 million times greater than the energy release in a chemical reaction involving deuterium and tritium (DT) molecules. This highlights the immense potential of fusion reactions for generating large amounts of energy.
What are the effects of fusion on the environment?
Fusion energy stands as one of the most environmentally friendly sources of energy. It distinguishes itself by producing no harmful atmospheric emissions, including CO2, which makes fusion a carbon-neutral energy solution. By avoiding greenhouse gas emissions, fusion energy plays a crucial role in mitigating the impact of global warming. Additionally, fusion fuel sources such as hydrogen and lithium are abundantly available in various regions worldwide, ensuring a sustainable and accessible supply for fusion reactions.
The Promise of Fusion Energy
Fusion has the extraordinary potential to generate 20 to 100 million times more energy than traditional fossil fuel reactions. Recent advancements in fusion research have sparked immense enthusiasm and curiosity in this field. Thanks to groundbreaking scientific discoveries, we have witnessed the creation of a nuclear reaction that produces more energy than it consumes. [2]
In a groundbreaking achievement, US scientists achieved a significant milestone in December 2022. For the first time ever, they successfully generated more energy from fusion than the amount of laser energy they utilized to fuel the experiment. This remarkable feat marks a major breakthrough in the quest for harnessing the immense power of fusion for clean and sustainable energy generation.
The Role of Simulations in Fusion Research
Plasma Physics
One of the most important challenges in fusion research is the complexity of the physics involved. Fusion plasmas exhibit a diverse array of phenomena that occur across a wide range of time and spatial scales. (3)
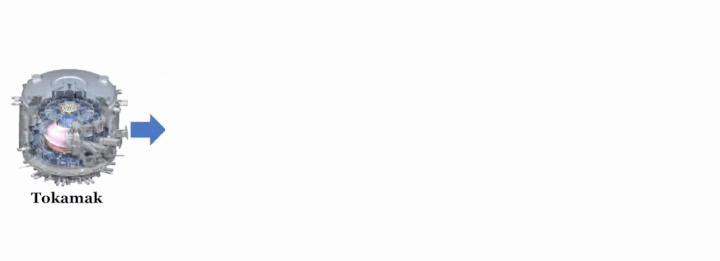
The figure above, as depicted by (3), illustrates how scientists utilize laser measurements to determine the plasma's pressure within a tokamak, providing valuable insights into its internal forces and dynamics. Additionally, the use of infrared cameras enables visualization and in-depth study of the plasma's thermal characteristics. In the realm of time and space scales, scientists encounter a diverse range of phenomena. At incredibly short time scales (approximately 10^-10 seconds), they observe wave propagation, which involves the transmission of electromagnetic waves through the plasma. This phenomenon holds significant importance in comprehending the plasma's microscopic behavior.
Computational Costs
Another important challenge in fusion research is the computational cost of simulations. Plasma researchers have developed high-fidelity models such as ELMFIRE, GYRO, and GENE, which simulate plasma behavior in large fusion devices. However, these gyro-kinetic codes require extensive computational resources, taking years on high-performance computing systems to simulate just a single second of plasma (5). In order to address this limitation, reduced models like TGLF and QuaLiKiz have been developed, which still require several hours on multiple processors for simulations.
To achieve significant speed-ups, surrogate models based on feed-forward neural networks have been introduced. These surrogate models, such as QLKNN and TGLF-NN, have enabled a remarkable reduction in simulation time, reaching seconds on a single processor. This represents an order of magnitude improvement in computational efficiency. These advancements in surrogate modeling have paved the way for real-time flight simulators that could be employed for control purposes in future fusion power plants.
Source [6]
ML Techniques for Accelerated Fusion Simulations
During the IAEA webinar in March 2023, Dr. M. Churchill, a representative from PPPL, delivered a presentation on the integration of machine learning (ML) techniques into fusion codes with the goal of accelerating fusion simulations. One particular code highlighted during the talk was the X-Point Included Gyrokinetic Code (XGC), a large-scale gyrokinetic code specifically designed for simulating turbulence and transport phenomena occurring in the tokamak edge.[3]
One of the challenges in the XGC code is how particles collide with each other. This is solved using something called the Fokker-Planck collision operator. However, when there are many different types of particles involved, this collision operator can slow down the simulations. In order to run more detailed simulations with lots of different particles, Dr. Churchill mentioned the need to make the collision operator faster. [3]
The images provided below display the impressive turbulence modeling abilities of the GENE (left side) and XGC codes ( the right side ), offering a glimpse into the intricate patterns and behaviors observed in the simulations. By observing the accompanying animation, it becomes apparent that the core region undergoes changes at a slower rate when compared to the edge region. This observation sheds light on the complex dynamics and behaviors exhibited by plasma in fusion systems, providing valuable insights into their behavior and operation.
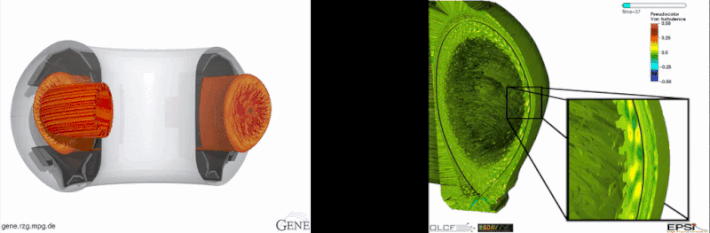
The tokamak edge holds great significance as it enables the prediction of heat loads on the wall, prevents wall damage caused by excessive heat, and contributes to achieving optimal performance within the edge region. These factors are vital in maximizing the core performance of the fusion process and ultimately attaining the desired fusion energy output. The presentation shed light on the advancements made in fusion research through the integration of ML techniques, paving the way for improved simulations and a deeper understanding of plasma behavior in fusion systems.
Conclusion
The integration of machine learning techniques, such as neural networks, has significantly enhanced our understanding and control of plasma behavior. By leveraging AI, we have gained deeper insights into the complex dynamics of fusion energy and have the potential to optimize the performance of fusion reactors. The convergence of AI and fusion energy presents a promising avenue for scientific innovation.
As we conclude this blog, we invite you to stay tuned for upcoming articles that will further explore the fusion energy revolution fueled by AI. Together, let us continue to unlock the true potential of AI and pave the way for a brighter and more sustainable future.
References
- Pukhov, A. (2014). Particle-In-Cell Codes for Plasma-based Particle Acceleration. Published by CERN in the Proceedings of the CAS-CERN Accelerator School: Plasma Wake Acceleration, Geneva, Switzerland, 23–29 November 2014.
- MIT Climate Portal. (2023). Fusion Energy. Retrieved [24/06/2023], from https://climate.mit.edu/explainers/fusion-energy
- ITU event (2023). AI for Good Webwinear with Dr. Michael Churchill et al. Retrieved from https://www.youtube.com/watch?v=zNn0NCcqc98&t=303s
- Dubus, G. D. (2014). From plain visualisation to vibration sensing: using a camera to control the flexibilities in the ITER remote handling equipment.
- Garbet, X., Idomura, Y., Villard, L., & Watanabe, T. H. (2010). Gyrokinetic simulations of turbulent transport. Nuclear Fusion, 50(4), 043002.
- Kai Nordlund et al (2021) Machine learning approaches to facilitate fusion research. Retrieved from https://www.youtube.com/watch?v=BU4kjWwjwrk